- Vaccine hesitancy is among the major threats to the effectiveness of vaccination programmes. This study aimed to report the trend in response to willingness to accept the COVID-19 vaccine between two waves of the local epidemic and examine differences among occupations. Two cross-sectional surveys were conducted online during the first wave (February) and third wave.
- Performance of the algorithm (black curve) and ophthalmologists (colored circles) for the presence of referable diabetic retinopathy (moderate or worse diabetic retinopathy or referable diabetic macular edema) on A, EyePACS-1 (8788 fully gradable images) and B, Messidor-2 (1745 fully gradable images).
Rates in AI/AN females were higher than rates in white females. Rates of adenocarcinoma of the central/distal stomach were significantly higher in AI/AN males than in whites for each region individually, with RRs ranging from 2 in the East to over 11 in Alaska, and for all regions combined (overall RR = 3.74, 95% CI 3.30–4.22).
San Francisco-based CircleCI, which develops continuous delivery automation software, today revealed that it closed a $56 million series D funding round led by Owl Rock Partners and Next Equity, with participation from existing investors Scale Venture Partners, Top Tier Capital, DFJ, Baseline Ventures, Industry Ventures, Heavybit, and Harrison Metal Capital. The capital infusion brings the startup’s total raised to $115.5 million following a $31 million series C round in January 2018, and CEO Jim Rose says the funding will further CircleCI’s “extensibility” and “agnosticism” capabilities and help to expand the company’s global presence.
“Our rapid pace of change over the last 18 months reflects our industry as a whole: software development is increasingly complex, fragmented, and difficult to map. CircleCI is in the unique position to help engineering organizations write better software and deliver value faster,” said Rose. “Our product strategy combines an agnostic, build anything, anywhere roadmap with a deep focus on lifting business value delivery of software everywhere.”
CircleCI — which was cofounded in 2011 by Allen Rohner and Paul Biggar and which counts among its customers Facebook, Coinbase, Sony, Kickstarter, GoPro, Spotify, Segment, and Percolate — develops a tool set that integrates with Bitbucket and GitHub to create builds the minute code is committed. It automatically tests those builds in containers or virtual machine and notifies teams if issues arise, and it deploys passing builds to target environments such as Docker images or virtual Linux, Android, or macOS machines.
Dev teams can define and orchestrate how execution runs and take advantage of Docker support to build images from registries. Moreover, they’re able to specify required compute and memory resources and tap caching options to speed up builds with save and restore points.
CircleCI’s SSH support lets managers or dev team members run jobs in local environments, and its security tools — which include LDAP for user management, audit logging, and full-level virtual machine isolation — shields code from illicit tampering. On the analytics side of things, an interactive visual dashboard collates metrics in one place, including the number of failed builds and the slowest tests.
CircleCI’s platform can be installed on a private server, and the company offers a managed cloud service that includes instant access to feature releases and automatic upgrades. No matter where it’s installed, CircleCI supports any language that builds on Linux or macOS, including C++, Javascript, .NET, PHP, Python, and Ruby.
“Every company today relies on software to continuously improve its products and business in order to keep up with consumers’ evolving needs and expectations,” said CircleCI chief technical officer Rob Zuber. “We believe humans should never have to wait on machines. This new round of funding will better allow us to provide developers flexibility and control to power their workflows seamlessly.”
It’s been a banner few years for CircleCI, which recorded an increase in the number of monthly jobs on its platform from 7 million to more than 30 million. CircleCI recently expanded its technology partner program (CircleCI Orbs) to 800 integrations across 45 partners (including Azure, Fastlane, and Slack) ahead of the launch of its v2 API, and it opened its first international office in Tokyo along with hubs in Boston and Denver. (Orbs, which launched eight months ago, allows teams and devs to share preferred configurations by packaging commands, executors, and jobs into lines of code.)
In other news, CircleCI brought aboard 75 new employees in the first six months of 2019, with projections to have a total of 300 by 2020. And it added to its C-suite with the promotions of Chitra Balasubramanian to chief financial officer, Jane Kim to chief revenue officer, and Erich Ziegler to chief marketing officer.
CircleCI says it’s already running Windows jobs for customers in addition to Linux, Docker, and macOS, and says that it intends to support additional git providers in the future (such as GitLab). It’s also testing a new user interface and improving its onboarding process, and it says it plans to preview a new usage-based pricing model that’ll gives teams per-job control over resource classes.
“When evaluating companies for investment, one quality I look for is how quickly a company can deliver value to the market,” said Scale Venture Partners partner Andy Vitus. “Scale led CircleCI’s Series B in 2016, and since then I’ve seen the company prove how effectively it improves developer throughput and velocity. We believe CircleCI is the DevOps standard for companies looking to accelerate their delivery pipeline while increasing quality.”
VentureBeat
VentureBeat's mission is to be a digital town square for technical decision-makers to gain knowledge about transformative technology and transact. Our site delivers essential information on data technologies and strategies to guide you as you lead your organizations. We invite you to become a member of our community, to access:- up-to-date information on the subjects of interest to you
- our newsletters
- gated thought-leader content and discounted access to our prized events, such as Transform
- networking features, and more
Automated assessment of intraoperative technical skill in capsulorhexis
Tae Soo Kim et al., IPCAI 2019, Rennes, France
PURPOSE:
Objective assessment of intraoperative technical skill is necessary for technology to improve patient care through surgical training. Our objective in this study was to develop and validate deep learning techniques for technical skill assessment using videos of the surgical field.
METHODS:
We used a data set of 99 videos of capsulorhexis, a critical step in cataract surgery. One expert surgeon annotated each video for technical skill using a standard structured rating scale, the International Council of Ophthalmology's Ophthalmology Surgical Competency Assessment Rubric:phacoemulsification (ICO-OSCAR:phaco). Using two capsulorhexis indices in this scale (commencement of flap and follow-through, formation and completion), we specified an expert performance when at least one of the indices was 5 and the other index was at least 4, and novice otherwise. In addition, we used scores for capsulorhexis commencement and capsulorhexis formation as separate ground truths (Likert scale of 2 to 5; analyzed as 2/3, 4 and 5). We crowdsourced annotations of instrument tips. We separately modeled instrument trajectories and optical flow using temporal convolutional neural networks to predict a skill class (expert/novice) and score on each item for capsulorhexis in ICO-OSCAR:phaco. We evaluated the algorithms in a five-fold cross-validation and computed accuracy and area under the receiver operating characteristics curve (AUC).
RESULTS:
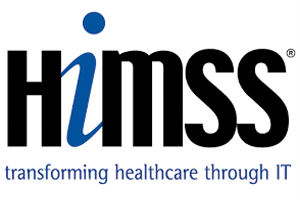
The accuracy and AUC were 0.848 and 0.863 for instrument tip velocities, and 0.634 and 0.803 for optical flow fields, respectively.
CONCLUSIONS:

Deep neural networks effectively model surgical technical skill in capsulorhexis given structured representation of intraoperative data such as optical flow fields extracted from video or crowdsourced tool localization information.
Evaluation of machine learning and deep learning algorithms to classify phases in cataract surgery procedures
Yu et al., JAMA Open, 2019
Importance
Competence in cataract surgery is a public health necessity, and videos of cataract surgery are routinely available to educators and trainees but currently are of limited use in training. Machine learning and deep learning techniques can yield tools that efficiently segment videos of cataract surgery into constituent phases for subsequent automated skill assessment and feedback.
Objective

To evaluate machine learning and deep learning algorithms for automated phase classification of manually presegmented phases in videos of cataract surgery.
Design, Setting, and Participants
This was a cross-sectional study using a data set of videos from a convenience sample of 100 cataract procedures performed by faculty and trainee surgeons in an ophthalmology residency program from July 2011 to December 2017. Demographic characteristics for surgeons and patients were not captured. Ten standard labels in the procedure and 14 instruments used during surgery were manually annotated, which served as the ground truth.
Exposures
Five algorithms with different input data: (1) a support vector machine input with cross-sectional instrument label data; (2) a recurrent neural network (RNN) input with a time series of instrument labels; (3) a convolutional neural network (CNN) input with cross-sectional image data; (4) a CNN-RNN input with a time series of images; and (5) a CNN-RNN input with time series of images and instrument labels. Each algorithm was evaluated with 5-fold cross-validation.
Main Outcomes and Measures
Accuracy, area under the receiver operating characteristic curve, sensitivity, specificity, and precision.
Results
Unweighted accuracy for the 5 algorithms ranged between 0.915 and 0.959. Area under the receiver operating characteristic curve for the 5 algorithms ranged between 0.712 and 0.773, with small differences among them. The area under the receiver operating characteristic curve for the image-only CNN-RNN (0.752) was significantly greater than that of the CNN with cross-sectional image data (0.712) (difference, −0.040; 95% CI, −0.049 to −0.033) and the CNN-RNN with images and instrument labels (0.737) (difference, 0.016; 95% CI, 0.014 to 0.018). While specificity was uniformly high for all phases with all 5 algorithms (range, 0.877 to 0.999), sensitivity ranged between 0.005 (95% CI, 0.000 to 0.015) for the support vector machine for wound closure (corneal hydration) and 0.974 (95% CI, 0.957 to 0.991) for the RNN for main incision. Precision ranged between 0.283 and 0.963.
Conclusions and Relevance
Time series modeling of instrument labels and video images using deep learning techniques may yield potentially useful tools for the automated detection of phases in cataract surgery procedures.
Structuring Expert Insights for Automated Coaching of Surgical Skills
Kim Roseolms Ai Ci Programs

Kim Roseolms Ai Ci Program
Bontrager et al., Association of Surgical Education Annual Meeting at 2019 Surgical Education Week, Chicago
Kim Roseolms Ai Ci Program Reviews
Emily Bontrager (Neuroscience) presented our preliminary work on qualitative data analysis of narratives obtained from expert surgeons while performing robot-assisted needle passing skill on a virtual reality training platform. Project Link
